What Is Industrial AI?
Industrial AI is the application of artificial intelligence (AI) technology to augment, enhance and optimize various digital initiatives and processes within asset-heavy industries. AI can improve efficiency, productivity, quality and decision-making in these industries by predicting outcomes and automating tasks across the manufacturing value chain from concept to design and all the way to aftermarket and end of life.
Enterprises that are integrating Industrial AI into their daily operations are fueling growth and boosting productivity. Reimagining product design using Industrial AI can profoundly impact time to market as well as aid product teams that are under more pressure than ever to accelerate design cycles and innovate. Enterprises can leverage Industrial AI in product design to differentiate from competitors and deliver a unique value proposition to customers.
Reimagining Product Design with AI
Companies must deliver highly customized, innovative products to market swiftly. Industrial AI can help optimize time to market for new products by analyzing trends and historical design and engineering data to help engineers generate new designs, track and monitor any issues that may arise across the product design value stream and drive collaboration across various stakeholders. The biggest challenge enterprises face in implementing AI in product design is curating, cleansing and contextualizing the historical engineering and design data into information and knowledge that can be used to build, train and refine the Industrial AI models.
The design process typically follows the tried-and-tested sequential approach:
- Ideate: identify a problem and come up with a concept.
- Create: build a rough prototype of the concept.
- Design: fill in the details with a comprehensive design.
- Validate: test the product to ensure it works.
- Manufacture: build the product at scale.
- Launch: release the product into the market.
The most common challenge of this approach is the extensive expertise and manual intervention needed at every stage to align product design with its intended purpose. The number of designs that can be conceived and frozen in the ideation process is limited by the time and human resources at hand. New digital methodologies include generative design, which is an iterative design process that uses software to generate outputs to fulfill a set of constraints iteratively and can be adjusted by a designer to eliminate such limitations. These design outputs, however, are devoid of past learnings. In other words, they do not use data. This is where Industrial AI solutions can help scale and redefine the product design process.
Enterprises that employ Industrial AI can foster a more sophisticated and seamless collaboration between software applications, data and engineers. To build Industrial AI solutions that augment the product design process, enterprises need to codify accumulated knowledge and lessons learned into semantic models so they can build enterprise-wide ontologies. An ontology organizes different kinds of data into classes and entities and shows the relationships between those entities and variants. Ontologies serve to ensure a common understanding of information and facilitate better data sense-making. This is imperative for Industrial AI solutions to effectively learn from data and improve process efficiencies.
How Does Industrial AI Work in Practice?
Reimaging the product development value stream is a good starting point to evaluate the art of the possible in product design.
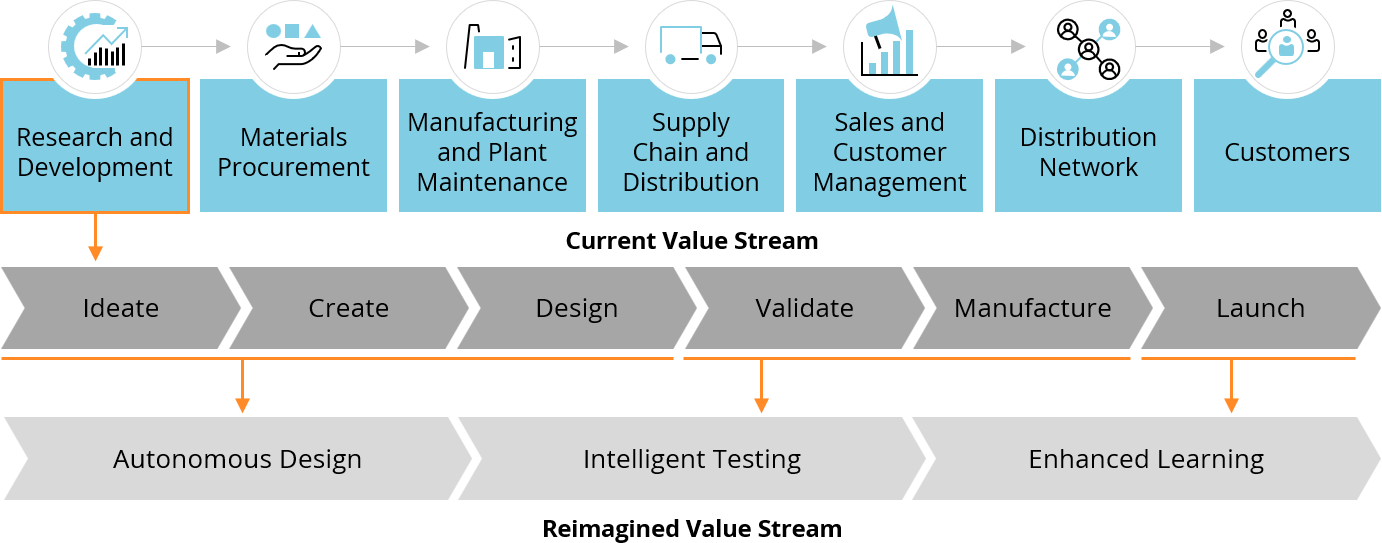
Figure 1: The Industrial AI Adoption Journey for Product Design
To leverage Industrial AI solutions for product design, enterprises can employ design assistant AI chatbots. These chatbots interact with data stored in repositories, accessing a data catalog for uniformity and human-friendly presentation. For example, a team designing a powertrain could ask the design assistant AI chatbot questions about the data, such as “What are similar designs in the past that can be reused or rehashed to meet current requirements?”.
The steps for using AI in product design would be as follows:
- Your data sits in a cloud data lake or warehouse (i.e., Bigquery, Snowflake, etc.).
- Metadata or a data catalog is referencing that data to make the data uniform, connected and “human friendly."
- An engineer asks a question to a chatbot, and it is run through an AI large language model (LLM) like ChatGPT
- The AI model uses the data catalog and its own knowledge to generate a query against the relevant dataset.
- Hopefully, an accurate answer is returned.
A major challenge lies in ensuring accuracy in step 4. This is where enterprises can enhance Industrial AI's performance by incorporating ontologies. We assume that the design assistant chatbot, in this case, can understand how the historical product design and engineering data works together to address your product engineering requirements, but it often misses nuances. Enterprises can add an ontology to make the AI model smarter and more accurate. If a subject matter expert crafts the ontology, the data map is even more powerful.
Ontologies refine and organize product design data so it can be leveraged by AI models. Though humans are still needed to check and approve the information, ontologies bring different data together by using common standards, making it easier to search and understand. Without an ontology, an AI model can get things wrong or use old information. Enterprises that have ontologies specific to their product and engineering needs will find that AI tools can conduct more reliable search queries.
How Do Generative Design Algorithms Help?
In traditional product design, even after coming up with a design area, connection points and constraint parameters, such as weight or torque, you spend hours calculating whether a proposed design would meet each of the engineering requirements. Conceptualization and validation of designs can be revolutionized with new generative design algorithms.
Generative design algorithms optimize designs not only for engineering parameters but also for commercial considerations, such as production costs and aesthetics. Generative design algorithms incorporate a wide range of constraints and showcase multiple design options swiftly. Engineers can input various requirements, and the Industrial AI then analyzes material applications and manufacturing principles based on past learnings. This not only streamlines engineering work but also unlocks new possibilities that previous time and budget constraints have limited. Automated simulations further expedite the design process, allowing engineers to focus on challenges requiring human intuition.
ISG helps asset-heavy enterprises understand and leverage Industrial AI solutions to redefine the product design process. Contact us to find out how we can get started.